41 learning to drive from simulation without real world labels
Learning to Drive from Simulation without Real World Labels Learning to Drive from Simulation without Real World Labels Alex Bewley, Jessica Rigley, Yuxuan Liu, Jeffrey Hawke, Richard Shen, Vinh-Dieu Lam, Alex Kendall Simulation can be a powerful tool for understanding machine learning systems and designing methods to solve real-world problems. Learning Interactive Driving Policies via Data-driven Simulation Data-driven simulators promise high data-efficiency for driving policy learning. When used for modelling interactions, this data-efficiency becomes a bottleneck: Small underlying datasets often lack interesting and challenging edge cases for learning interactive driving. We address this challenge by proposing a simulation method that uses in ...
Learning to Drive from Simulation without Real World Labels Abstract: Simulation can be a powerful tool for under-standing machine learning systems and designing methods to solve real-world problems. Training and evaluating methods purely in simulation is often "doomed to succeed" at the desired task in a simulated environment, but the resulting models are incapable of operation in the real world.
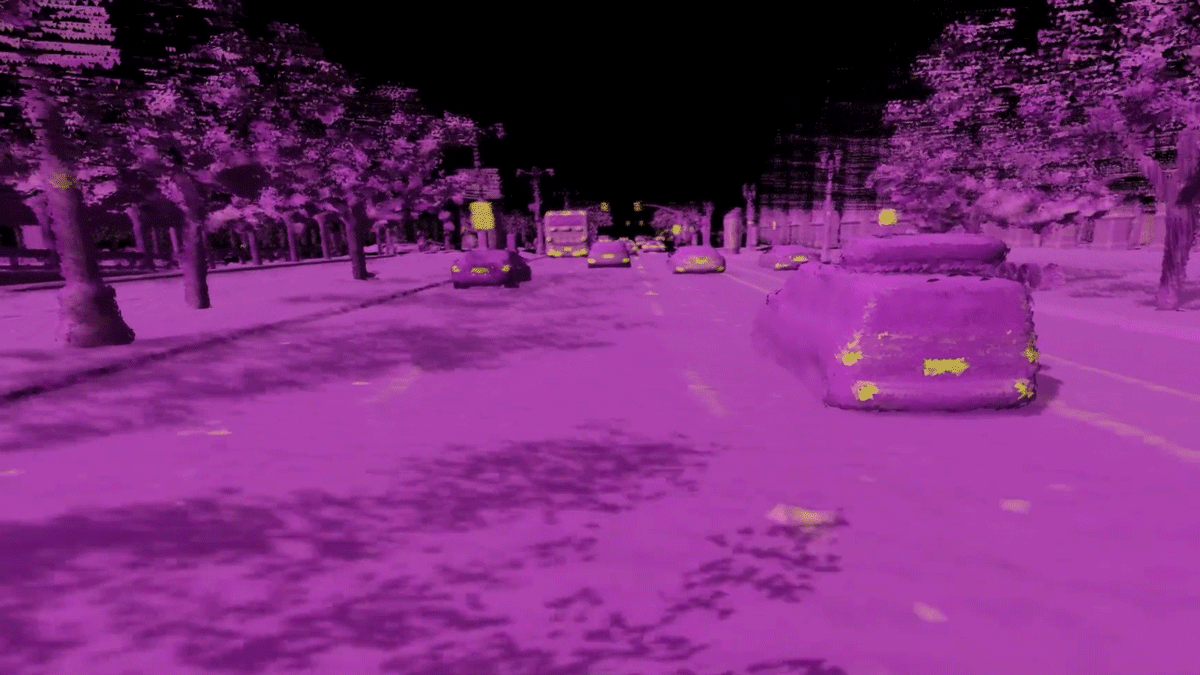
Learning to drive from simulation without real world labels
Learning to Drive from Simulation without Real World Labels Simulation can be a powerful tool for understanding machine learning systems and designing methods to solve real-world problems. Training and evaluating methods purely in simulation is often "doomed to succeed" at the desired task in a simulated environment, but the resulting models are incapable of operation in the real world. Learning to Drive from Simulation without Real World Labels Simulation can be a powerful tool for under-standing machine learning systems and designing methods to solve real-world problems. Training and evaluating methods purely in simulation is often "doomed to succeed" at the desired task in a simulated environment, but the resulting models are incapable of operation in the real world. Here we present and evaluate a method for transferring a ... Sim2Real: Learning to Drive from Simulation without Real World Labels See the full sim2real blog: drive on real UK roads using a model trained entirely in simulation.Research paper: ....
Learning to drive from simulation without real world labels. Learning to Drive from Simulation without Real World Labels Learning to Drive from Simulation without Real World Labels Authors: Alex Bewley Queensland University of Technology Jessica Rigley University of Cambridge Yuxuan Liu Jeffrey Hawke Wayve No... 论文笔记 Learning to Drive from Simulation without Real World Labels 文章对自己的贡献进行了总结:. 1、We present the first example of an end-to-end driving policy transferred from a simulation domain with control labels to an unlabelled real-world domain. 2、利用模拟器,我们可以学习到超越在真实世界中常见驾驶分布的策略,消除了对多个摄像头或者数据增强 ... Learning to Drive from Simulation without Real World Labels Learning to Drive from Simulation without Real World Labels Alex Bewley, Jessica Rigley, Yuxuan Liu, Jeffrey Hawke, Richard Shen, Vinh-Dieu Lam, Alex Kendall The authors are with Wayve in Cambridge, UK. Abstract Simulation can be a powerful tool for understanding machine learning systems and designing methods to solve real-world problems. (video) Sim2Real: Learning to Drive from Simulation without Real World ... See the full sim2real blog: We drive on real UK roads using a model trained entirely in ...
Learning to Drive from Simulation without Real World Labels Learning to Drive from Simulation without Real World Labels By Alex Bewley, Jessica Rigley, Yuxuan Liu, Jeffrey Hawke, Richard Shen, Vinh-Dieu Lam and Alex Kendall Get PDF (3 MB) Abstract Simulation can be a powerful tool for understanding machine learning systems Learning to Drive from Simulation without Real World Labels Learning to Drive from Simulation without Real World Labels A. Bewley, J. Rigley, +4 authors Alex Kendall Published 10 December 2018 Computer Science 2019 International Conference on Robotics and Automation (ICRA) Simulation can be a powerful tool for under-standing machine learning systems and designing methods to solve real-world problems. [...] PDF Learning to Drive from Simulation without Real World Labels - arXiv Learning to Drive from Simulation without Real World Labels Alex Bewley, Jessica Rigley, Yuxuan Liu, Jeffrey Hawke, Richard Shen, Vinh-Dieu Lam, Alex Kendall Abstract—Simulation can be a powerful tool for under-standing machine learning systems and designing methods to solve real-world problems. Training and evaluating methods Learning to Drive from Simulation without Real World Labels Learning to Drive from Simulation without Real World Labels Authors: Alex Bewley Queensland University of Technology Jessica Rigley University of Cambridge Yuxuan Liu Jeffrey Hawke Wayve Abstract...
Learning to Drive from Simulation Without Real World Labels Learning to Drive from Simulation Without Real World Labels; Segmentation and Deconvolution of Fluorescence; Mastering Openframeworks: Creative Coding Demystified; Image Evolution Using 2D Power Spectra; Noisy Gradient Meshes Procedurally Enriching Vector Graphics Using Noise; Better Gradient Noise; Noises Jaanus Jaggo Noise; Fast High-Quality ... Simulation Training, Real Driving | Wayve Our autonomous car drove on real UK roads by learning to drive solely in simulation. Our procedurally generated simulation environment. Our algorithm trained in simulation, driving in the real-world. Leveraging simulation is a powerful approach to gaining experience in situations which are expensive, dangerous or rare in the real world. Sim2Real: Learning to Drive from Simulation without Real World Labels See the full sim2real blog: drive on real UK roads using a model trained entirely in simulation.Research paper: .... Learning to Drive from Simulation without Real World Labels Simulation can be a powerful tool for under-standing machine learning systems and designing methods to solve real-world problems. Training and evaluating methods purely in simulation is often "doomed to succeed" at the desired task in a simulated environment, but the resulting models are incapable of operation in the real world. Here we present and evaluate a method for transferring a ...
Learning to Drive from Simulation without Real World Labels Simulation can be a powerful tool for understanding machine learning systems and designing methods to solve real-world problems. Training and evaluating methods purely in simulation is often "doomed to succeed" at the desired task in a simulated environment, but the resulting models are incapable of operation in the real world.
Post a Comment for "41 learning to drive from simulation without real world labels"